How AI Drug Repurposing is Breathing New Life into Shelved Compounds
The pharmaceutical industry is notorious for its high costs and prolonged timelines in drug development. On average, bringing a new drug to market can take over a decade and cost billions of dollars. Despite these massive investments, the attrition rate remains alarmingly high, with a significant majority of drug candidates failing in late-stage clinical trials due to unforeseen side effects or lack of efficacy in humans. This stark reality has prompted a search for innovative strategies to make drug development more efficient and successful.
One such strategy gaining significant traction is AI-driven drug repurposing. By leveraging artificial intelligence and machine learning, researchers and pharmaceutical companies can identify new therapeutic uses for existing compounds, effectively breathing new life into shelved drugs that were previously deemed ineffective for their original indications. This approach not only circumvents the need for extensive preclinical and early-stage clinical trials but also accelerates the development process and reduces associated costs.
Finding new therapeutic applications for existing compounds is of paramount importance in today’s medical landscape. With the growing need for effective treatments across a variety of diseases, AI-driven drug repurposing offers a promising avenue to address these demands. This innovative approach can unlock the untapped potential of shelved compounds, providing new hope for patients and revolutionizing the pharmaceutical industry.
The Traditional Drug Development Process
The traditional drug development process is a lengthy and expensive journey that involves several stages, each fraught with challenges and potential points of failure. The process begins with the discovery phase, where researchers identify potential drug candidates through laboratory studies and preclinical testing. This phase alone can take several years and requires substantial financial investment.
Once a promising compound is identified, it moves into preclinical studies, where it is tested in animal models to assess its safety and efficacy. If the compound shows potential, it progresses to clinical trials, which are conducted in three phases. Phase I trials involve a small group of healthy volunteers to evaluate safety and dosage. Phase II trials expand to a larger group of patients to assess efficacy and side effects. Finally, Phase III trials involve thousands of patients to confirm efficacy, monitor side effects, and compare the drug to standard treatments.
Despite the rigorous testing, the attrition rate for new drug candidates is alarmingly high. Only about 10% of drugs that enter clinical trials ultimately receive regulatory approval. This high failure rate, combined with the lengthy timelines and substantial costs, creates a significant financial burden on pharmaceutical companies. The average cost of developing a new drug can exceed $2.6 billion, factoring in the expenses of failed trials.
Given these challenges, there is an urgent need for alternative strategies that can improve the efficiency and success rates of drug development. AI-driven drug repurposing presents a viable solution, offering a more targeted and cost-effective approach to identifying new therapeutic uses for existing compounds. By bypassing some of the most time-consuming and expensive stages of traditional drug development, AI-driven strategies can significantly shorten the time to market and reduce the financial risks associated with drug discovery.
The Role of AI and Machine Learning in Drug Repurposing
Artificial intelligence (AI) and machine learning (ML) are transforming the landscape of drug repurposing by providing powerful tools to analyze vast and complex datasets. These technologies can process and interpret data far beyond human capabilities, uncovering hidden patterns and correlations that might otherwise go unnoticed. In the context of drug repurposing, AI and ML techniques are particularly valuable for analyzing molecular structures, biological pathways, and clinical outcomes to identify new therapeutic uses for existing compounds.
AI-driven drug repurposing starts with the integration of diverse datasets, including genomic information, chemical properties of drugs, patient health records, and outcomes from previous clinical trials. Machine learning algorithms are then employed to sift through this data, learning from known examples of drug interactions and responses. These algorithms can identify relationships between drugs and biological targets, predict how a drug might interact with different types of cells or tissues, and forecast potential therapeutic applications.
One of the most significant advantages of using AI and ML in drug repurposing is their ability to predict new therapeutic applications for previously shelved compounds. Many compounds that showed promise in early development stages were eventually abandoned due to lack of efficacy for their intended indications or unforeseen side effects. However, these compounds still hold therapeutic potential that may be applicable to other diseases or conditions. AI and ML can re-evaluate these shelved drugs by comparing their molecular structures and mechanisms of action against a vast array of disease models and biological pathways.
For instance, AI can simulate how a shelved drug interacts with various cancer cell types, taking into account the unique genetic and molecular characteristics of each type. By identifying these interactions, AI can suggest alternative uses for the drug that were not previously considered. This approach not only maximizes the value of existing compounds but also accelerates the drug development process, as repurposed drugs can bypass some of the early-stage trials required for new drug candidates.
How AI Drug Repurposing is Breathing New Life into Shelved Compounds at Notable Labs
Notable Labs, a clinical-stage predictive therapeutics company, is at the forefront of utilizing AI and machine learning to breathe new life into shelved compounds like volasertib. By leveraging their proprietary Predictive Medicine Platform (PMP), Notable Labs has developed an innovative approach that goes beyond traditional genomics-based precision medicine.
The PMP harnesses the power of AI and machine learning algorithms to analyze the functional response of a patient's cancer cells to various drug treatments. This advanced platform integrates computational models with robotic automation to simulate the complex interactions within the tumor microenvironment, including cancer cells, immune cells, and surrounding tissues. By doing so, the PMP can accurately predict which patients are most likely to respond to a particular therapy.
For volasertib, Notable Labs' PMP identified a subset of AML patients who were highly sensitive to the drug, with a predicted response rate significantly higher than the standard of care. This crucial insight has allowed Notable Labs to optimize patient selection and design a more targeted Phase 2 clinical trial. By focusing on patients who are most likely to benefit from volasertib, Notable Labs aims to achieve better clinical outcomes and increase the likelihood of regulatory approval.
The advantages of this AI-driven drug repurposing approach extend beyond just identifying responsive patients. By leveraging existing safety and pharmacology data from previous clinical trials, Notable Labs can streamline the development process, reducing both timelines and costs. This predictive capability also holds the promise of improving clinical trial success rates, as the likelihood of identifying effective treatments for specific patient populations is significantly enhanced.
In essence, Notable Labs exemplifies how AI and machine learning can transform the landscape of drug development. By repurposing shelved compounds and uncovering new therapeutic applications, they are paving the way for more efficient, cost-effective, and personalized treatment options for patients in urgent need. This innovative approach not only revitalizes previously abandoned drugs but also brings hope to the ongoing battle against devastating diseases like cancer.
Advantages of AI-Driven Drug Repurposing
Utilizing Existing Safety and Pharmacology Data to Streamline Development
One of the primary advantages of AI-driven drug repurposing is the ability to leverage existing safety and pharmacology data from previous clinical trials. This wealth of pre-existing information significantly reduces the time and resources required for the early stages of drug development. By using data that has already been thoroughly vetted, researchers can bypass some of the initial safety trials, allowing them to focus on efficacy studies and specific patient populations more quickly.
Reduction in Development Timelines and Associated Costs
Traditional drug development is notorious for its lengthy and costly process, often taking over a decade and billions of dollars to bring a new drug to market. AI-driven drug repurposing offers a more efficient alternative. By predicting new uses for existing compounds, researchers can cut down the development timeline drastically. This efficiency translates to significant cost savings, as fewer resources are needed for preliminary research and development phases. This expedited process not only benefits pharmaceutical companies but also accelerates the availability of potentially life-saving treatments for patients.
Improvement in Clinical Trial Success Rates Through Better Patient Selection
AI and machine learning algorithms excel at analyzing complex datasets to identify patterns and correlations that might be missed by traditional methods. This capability is particularly valuable in improving patient selection for clinical trials. By pinpointing the subsets of patients most likely to respond to a repurposed drug, AI can enhance the precision of clinical trials. This targeted approach increases the likelihood of successful outcomes, reducing the risk of trial failure due to poor patient selection. Ultimately, this leads to more effective treatments reaching the market more quickly.
Potential for Regulatory Approval Acceleration
The ability to repurpose drugs with established safety profiles also holds the potential to expedite the regulatory approval process. Regulatory agencies, such as the FDA, prioritize drugs that have demonstrated safety in previous trials, allowing for a more streamlined review process. When combined with compelling efficacy data from AI-driven predictions, repurposed drugs can potentially receive faster regulatory approval. This accelerated path to market not only benefits pharmaceutical companies by reducing the financial risks associated with drug development but also brings much-needed treatments to patients in a more timely manner.
AI-driven drug repurposing represents a paradigm shift in pharmaceutical research, offering a strategic approach to maximize the therapeutic potential of existing compounds. By harnessing the power of AI and leveraging existing data, this innovative method streamlines development, reduces costs, and improves clinical outcomes, paving the way for a new era of personalized and efficient drug therapies.
Challenges and Considerations
Ethical and Practical Challenges in AI-Driven Drug Repurposing
While AI-driven drug repurposing holds immense potential, it is not without its ethical and practical challenges. One significant ethical concern is the transparency of AI algorithms. The 'black box' nature of many machine learning models can make it difficult to understand how they arrive at specific conclusions. This lack of transparency can lead to skepticism among clinicians and regulators, who may be hesitant to rely on AI-driven predictions without a clear understanding of the underlying mechanisms.
Additionally, there are practical challenges related to patient consent and data privacy. AI-driven research often requires access to vast amounts of patient data, which raises concerns about how this data is collected, stored, and used. Ensuring that patient data is handled ethically and securely is paramount, and researchers must navigate complex regulations to protect patient privacy while still harnessing the power of AI.
Need for Robust Computational Infrastructure and Data Management
AI-driven drug repurposing relies heavily on computational power and sophisticated data management systems. High-performance computing infrastructure is necessary to process and analyze the large datasets involved in this research. This requirement can pose a significant barrier for smaller research institutions or startups that may not have the resources to invest in such infrastructure.
Effective data management is also crucial. Integrating diverse data types—from genomic and proteomic data to clinical trial results—requires advanced data management systems capable of handling large-scale, multi-dimensional datasets. Ensuring the quality, consistency, and interoperability of these data sources is essential for generating reliable AI predictions. This need for robust infrastructure and data management underscores the importance of collaboration between technology developers, researchers, and healthcare institutions.
Regulatory and Validation Hurdles
Navigating the regulatory landscape is another significant challenge for AI-driven drug repurposing. Regulatory bodies like the FDA have established rigorous standards for drug approval, which are primarily designed for traditional drug development processes. Adapting these frameworks to accommodate AI-driven approaches requires substantial effort and collaboration between regulatory agencies and researchers.
Validation of AI predictions presents its own set of challenges. While AI can predict potential therapeutic applications, these predictions must be validated through rigorous clinical trials to ensure their safety and efficacy. This process can be time-consuming and expensive, potentially offsetting some of the time and cost savings achieved through AI-driven repurposing. Moreover, the dynamic and evolving nature of AI algorithms means that continuous validation and updating of models are necessary, adding another layer of complexity to the regulatory process.
Despite these challenges, the potential benefits of AI-driven drug repurposing make it a promising avenue for future drug development. By addressing these ethical, practical, and regulatory hurdles, the pharmaceutical industry can harness the full potential of AI to bring new life to shelved compounds and deliver more effective treatments to patients.
Future Directions
Summary of the Transformative Impact of AI-Driven Drug Repurposing
AI-driven drug repurposing is poised to revolutionize the pharmaceutical industry by breathing new life into shelved compounds and identifying novel therapeutic uses for existing drugs. This innovative approach addresses the high costs and lengthy timelines associated with traditional drug development, offering a more efficient and cost-effective pathway to bringing new treatments to market. By leveraging the power of AI and machine learning, researchers can uncover previously overlooked opportunities, providing new hope for patients with unmet medical needs.
Emphasis on the Shift Towards Innovative and Efficient Drug Development Strategies
The integration of AI in drug repurposing represents a significant shift towards more innovative and efficient drug development strategies. This approach not only accelerates the drug discovery process but also enhances the precision of treatment by identifying patient populations most likely to benefit from specific therapies. As AI technologies continue to advance and become more integrated into pharmaceutical research, the potential for transformative impact on drug development and patient care will only grow.
Future Potential of AI in Revolutionizing Pharmaceutical Research and Providing New Hope for Patients
Looking ahead, the future potential of AI in revolutionizing pharmaceutical research is immense. By expanding the applications of AI-driven drug repurposing beyond oncology, advancing AI and ML algorithms, and fostering collaborative efforts, the pharmaceutical industry can unlock new therapeutic possibilities and improve patient outcomes. AI-driven drug repurposing offers a promising solution to some of the most pressing challenges in drug development, paving the way for a more personalized and effective approach to medicine. As we continue to explore and harness the power of AI, we move closer to a future where innovative treatments are developed more efficiently, providing new hope for patients worldwide.
Conclusion
The pharmaceutical industry faces high costs and lengthy timelines in drug development, with many candidates failing in late-stage trials. AI-driven drug repurposing offers a promising solution by leveraging artificial intelligence and machine learning to identify new therapeutic uses for existing compounds. This approach circumvents extensive preclinical trials, accelerates development, and reduces costs, making it a valuable strategy for finding effective treatments.
By utilizing AI to analyze vast datasets, researchers can uncover new applications for shelved compounds, providing new hope for patients and transforming the pharmaceutical landscape. This innovative method enhances precision in treatment, improves clinical trial success rates, and expedites regulatory approval. As AI technology advances and integrates more deeply into pharmaceutical research, the potential for developing personalized, effective treatments increases, offering significant benefits for patients and the healthcare industry.
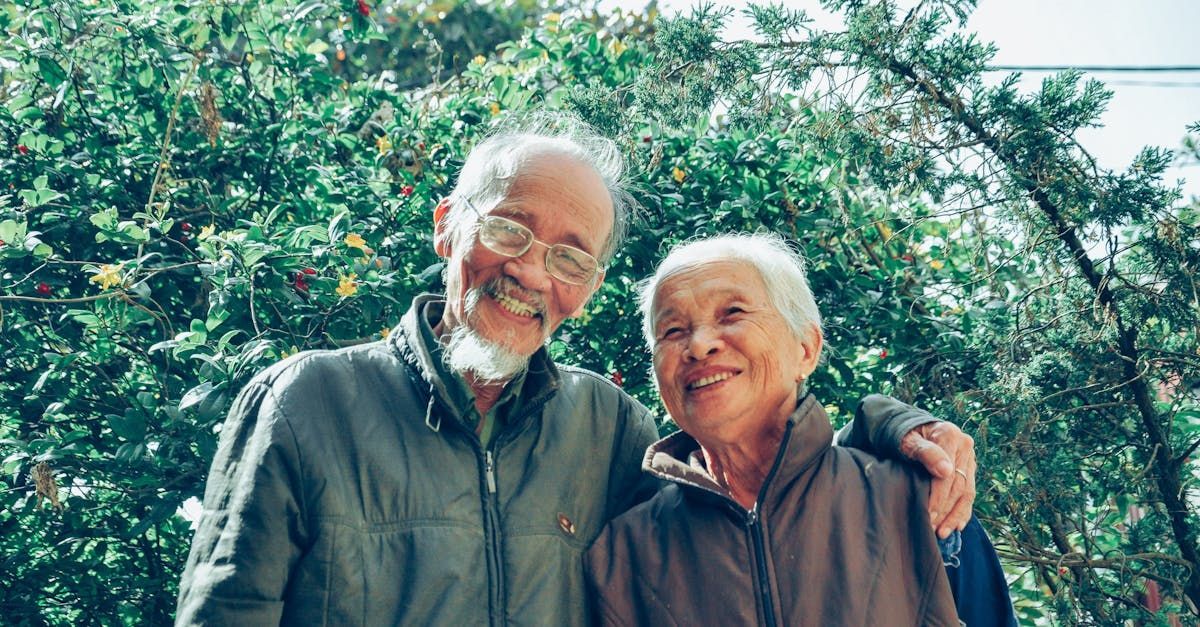
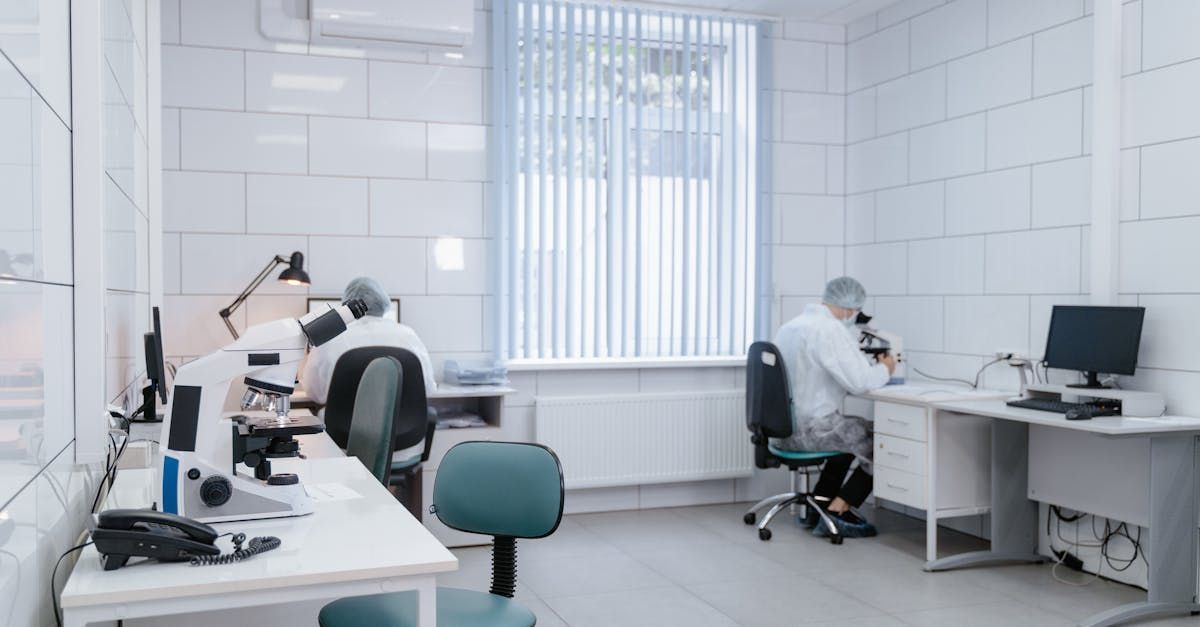